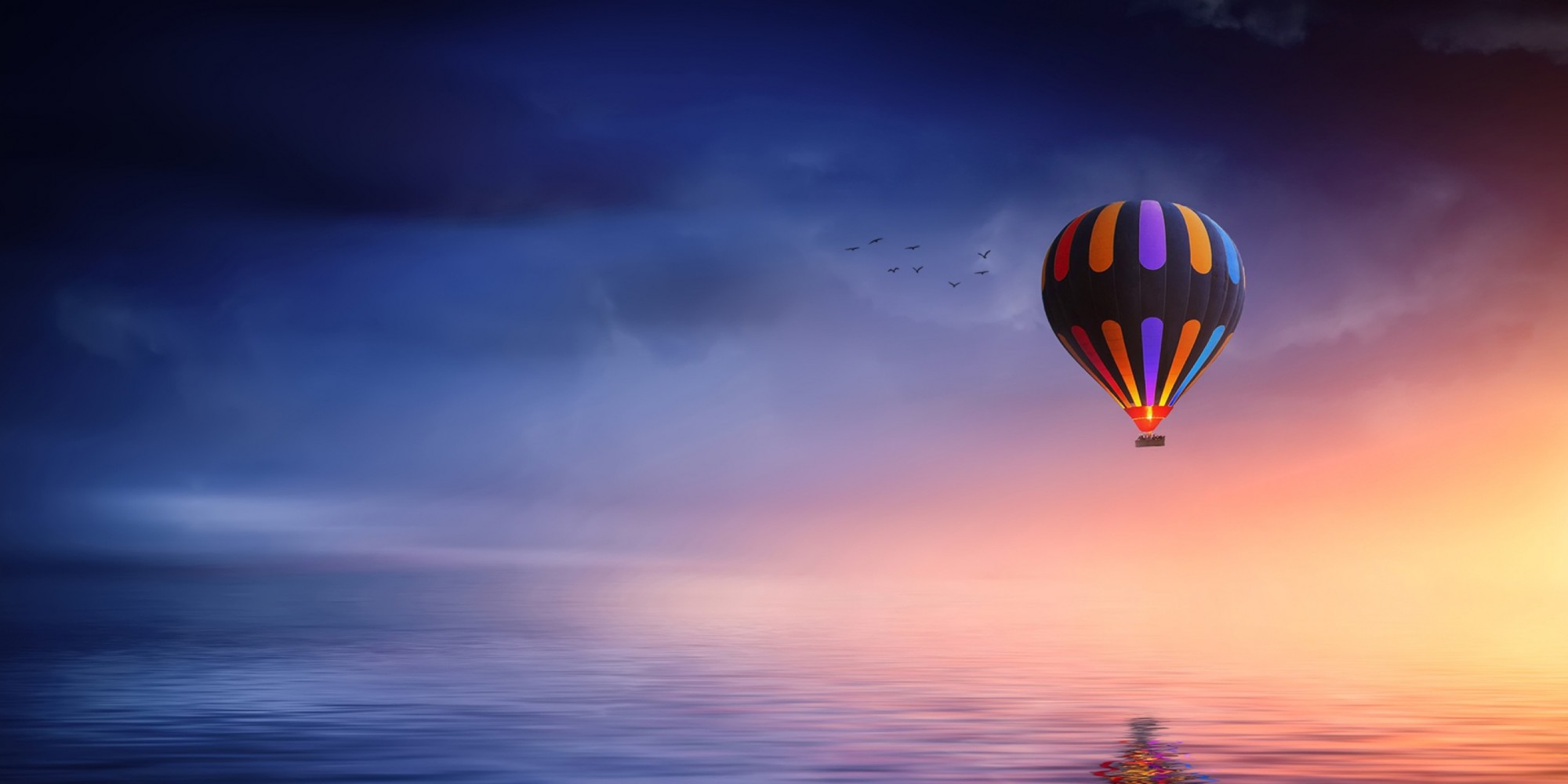
3 Common Data Lake Misconceptions
The enterprise data lake is now well past its infancy: more than a quarter of all organizations have a data lake in production. However, with maturity comes new findings, criticisms, and data lake misconceptions — with headlines like “Data lakes will need to demonstrate business value or die”.
Many of the criticisms of data lakes are just flat out untrue, so I’m here to set the record straight with three common misconceptions about data lakes, debunked:
They are a replacement to data warehouses
Some people call data lakes the next generation of data warehousing, or simply data warehouse 2.0. However, this couldn’t be farther from the truth. While both technologies at the core are data storage repositories capable of processing, manipulating, and securing data, they are both meant for different purposes, and thus are most efficient when coexisting with one another.
A key difference is that data lakes can store all and any type of data, whether it be structured, unstructured, or semi-structured, while data warehouses can only store structured data. In layman’s terms, Pentaho CTO James Dixon (credited with coining “data lake”), famously said “a data mart or data warehouse is akin to a bottle of water — cleansed, packaged and structured for easy consumption — while a data lake is more like a body of water in its natural state.”
Because data lakes are meant to store and process all types of data, they are ideal for data science and big data analytics projects, while data warehouses make more sense for primary applications where security and performance are valued most. Together, data lakes and data warehouses help enterprises manage their data and make better data-driven decisions.
Data lakes are not secure
Here’s another to add to the data lake misconceptions list: Key comparison of data lakes vs data warehouses is security — while data warehouses have been around longer and are considered much more mature for securing data, data lakes can be just as secure — the key is not in the technology, rather the overall data management strategy.
To secure your data lake, you must understand the data lake pipeline, from ingestion to analysis, and implement the appropriate data governance and security strategies accordingly.
Data lakes eventually become “data swamps”
Since data lakes ingest all and any types of data, organizations often worry that their data lakes will turn into “data swamps”, or huge repositories full of disorganized, poorly managed data. The key to avoiding a data swamp is to ensure the proper implementation of a fully featured Information Lifecycle Management strategy for your data lake.
Utilizing tools to ensure data can be classified on ingestion or creation and the correct retention policies applied down to the individual record basis. This ensures that the data is not retained past its usefulness and its purge from the system is fully audited on removal. Along with data retention, the data lake should be configured to support ‘Data Tiering’ to enable enterprises to store their data in the layer appropriate to its usage and long term life expectancy.
The Solix CDP’s object workbench and data governance workbench are built with all of the information lifecycle management tools necessary to prevent your data lake from turning into a data swamp, better preparing your data for advanced tasks like big data analytics, machine learning, and artificial intelligence.
Conclusion
Just like the adoption of any other technology in the enterprise, a successful data lake implementation does not stop at “if you build it, they will come”. For a data lake to become successful, enterprises must create a thorough data management strategy, and fortunately, there are many solutions readily available to help enterprises do so.