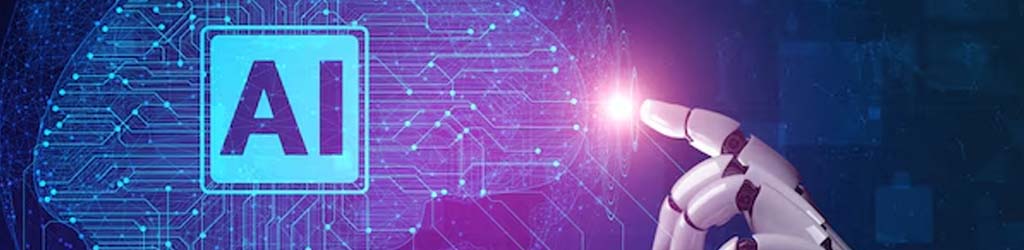
Agentic AI and Generative AI: Understanding the Difference
Our perception of AI has dramatically evolved over the last few decades. Where we once imagined AI as an all-powerful, terminator-like machine that could potentially go rogue, we now interact with intelligent systems that seamlessly integrate into our everyday lives. From Hollywood-fueled fantasies of sentient robots to the practical, versatile AI tools we use today, the journey of artificial intelligence has been nothing short of remarkable.
The technological landscape now has two particularly intriguing concepts: Generative AI and Agentic AI. While they might sound like complex technological terms, they represent distinctly different approaches to artificial intelligence that reshape how we understand machine capabilities.
Understanding Generative AI
Generative AI is perhaps the more familiar concept to most people. At its core, generative AI refers to systems that can create new content based on the data they’ve been trained on. This could include text generation (like writing articles, blogs, stories, or even code), image creation, music composition, or video creation.
In a very naive sense, generative AI is similar to a very sophisticated mimicry machine—something that can learn patterns from vast amounts of training and then produce similar but unique outputs that reflect those learning patterns. Large language models like GPT-4, Llama 3, Claude 3.5 Sonnet, and image generators like DALL-E and CM3Leon are prime examples of generative AI.
Exploring Agentic AI
Agentic AI represents a more complex and dynamic approach to artificial intelligence. Unlike generative AI, which primarily focuses on content creation, agentic AI is designed to make autonomous decisions, pursue complex goals, adapt to changing environments, and take proactive instead of reactive steps to achieve specific objectives. An agentic AI system doesn’t just generate content; it strategizes, plans, and executes multi-step tasks with autonomy beyond simple pattern recognition.
Here are some key capabilities of agentic AI:
- Task decomposition and planning
- Problem-solving and reasoning
- Learning and Adaptation
- Autonomous Decision-making
- Multi-modal interaction
Differences between Agentic AI and Generative AI
Aspect | Generative AI | Agentic AI |
---|---|---|
Primary Function | Content creation and generation | Goal-directed autonomous action |
Interaction Mode | Reactive, prompt-response | Proactive, strategic problem-solving |
Task Execution | Follows direct instructions | Breaks down complex tasks independently |
Decision Making | Limited, based on prompt context | Dynamic, with reasoning and adaptation |
Autonomy Level | Low (depends on specific input) | High (can initiate and drive processes) |
Key Capabilities | Producing text, images, code pattern recognition, summarization, translation | Multi-step planning, self-reflection, error correction, context-maintenance |
Example Behaviors | Answering questions, generating content | Setting goals, investigating problems, iterating solutions |
Learning Approach | Static knowledge from training data | Adaptive learning from interactions and feedback |
Complexity of Tasks | Primarily linear, single-step tasks | Complex, multi-stage objectives |
Practical Examples
- A content writer using GPT to draft blog posts
- An artist generating unique images with DALL-E
- A musician exploring AI-composed music
Generative AI in Action
- An AI research assistant that can independently design and run scientific experiments
- A financial trading system that adapts investment strategies in real-time
- An autonomous vehicle making complex navigation decisions
The Convergence
Interestingly, the lines between generative and agentic AI are not always clear-cut. Many modern AI systems incorporate elements of both, creating hybrid models that can generate content and make autonomous decisions.
Closing Thoughts
While generative AI excels at creating content and agentic AI focuses on autonomous problem-solving, both represent exciting frontiers in artificial intelligence. As these technologies continue to evolve, we can expect more sophisticated, versatile AI systems that blend generation and agency in unprecedented ways.
The future of AI is not just about creating or copying—it’s about understanding, reasoning, and independently working towards complex objectives.
About the Author
Hello there! I am Haricharaun Jayakumar, a senior executive in product marketing at Solix Technologies. My primary focus is on data and analytics, data management architectures, enterprise artificial intelligence, and archiving. I have earned my MBA from ICFAI Business School, Hyderabad. I drive market research, lead-gen projects, and product marketing initiatives for Solix Enterprise Data Lake and Enterprise AI. Apart from all things data and business, I do occasionally enjoy listening to and playing music. Thanks!