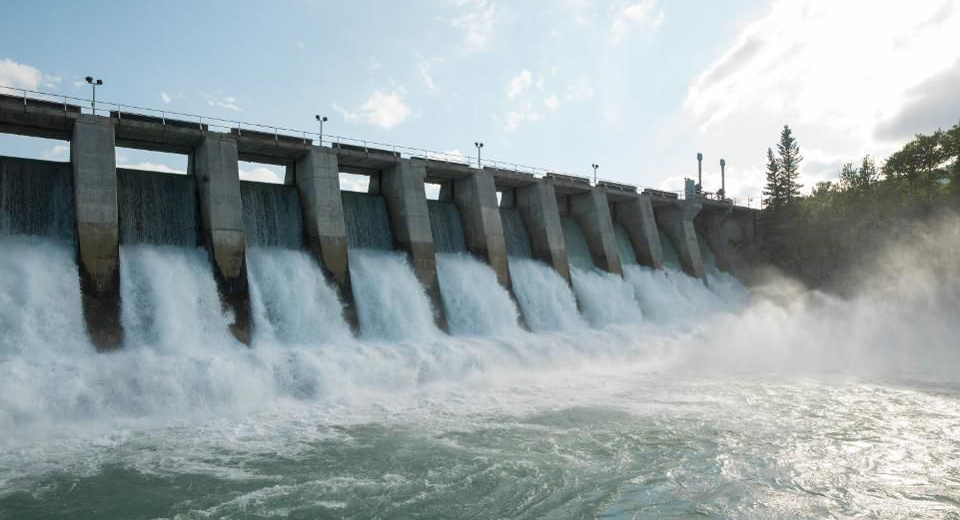
How To fill your data lakes and not lose control of the data
This post on data lakes was originally featured on Forbes.
Data lakes are everywhere now that cloud services make it so easy to launch one. Secure cloud data lakes store all the data you need to become a data-driven enterprise. And data lakes break down the canonical data structures of enterprise data warehouses, enabling users to describe their data better, gain better insights and make better decisions.
Data lake users are data-driven. They demand historical, real-time and streaming data in huge quantities. They browse data catalogs, prefer text search, and use advanced analytics, machine learning (ML) and artificial intelligence (AI) to drive digital transformation into the business. But where exactly does all the data come from?
The complexity of compliance and governance in data lakes
Filling data lakes is a complex process that must be done properly to avoid costly data preparation and compliance breakdowns. Data is collected from everywhere, and ingestion involves high volumes of data from IoT, social media, file servers, and structured and unstructured databases. Such large-scale data exchange poses significant data availability and data governance challenges.
Big data governance shares the same disciplines as traditional information governance, including data integration, metadata management, data privacy and data retention. But one important challenge is how to achieve centralized compliance and control over the vast amounts of data traversing multicloud networks of distributed data lakes.
And there is a sense of urgency. As digital transformation becomes a priority, data governance, data security and compliance must always be in place. Recently passed laws, specifically GDPR and CCPA, require robust data privacy controls, including “the right to be forgotten.” For many organizations, such compliance is a real challenge, even when it comes to answering the seemingly simple question, “Do you know where your data is?”
Federated Data Governance
One solution is a federated data governance model. Federated data governance solves the centralized versus decentralized dilemma. By establishing compliance controls at the point of data ingestion, information life cycle management (ILM) policies may be applied to classify and govern data throughout its life cycle. As high volumes of data move from databases and file servers and transform into cloud-based object storage, policy-driven compliance controls are needed like never before.
As a best practice to set up federated data governance, compliance policies and procedures should be standardized across the enterprise. Proper data governance involves business rules that are followed hard and fast. “Comply or explain” systems lead to distrust by audit authorities and require rigorous follow-up to ensure proper remedies are consistently applied. Once noncompliant data is released to the network, recall may not be possible.
Enterprise Data Lakes
An enterprise data lake is the centerpiece of the interconnected data fabric. Enterprise data lakes ingest data, prepare it for processing and provide a federated data governance framework to manage the data throughout its life cycle. Centralized, policy-driven data governance controls ensure compliant data is available for decentralized data lake operations.
Enterprise data lakes also speed up data ingestion. Centralized connections to import data from structured, semi-structured, unstructured and siloed S3 object stores simplify compliance control. Whether the data arrives as a simple “copy” or more complicated “move” function (for archiving), centralized ingestion enables data to be cataloged, labeled, transformed and governed with ILM and retention plans. As data is classified during ingestion, centralized security management and access control become possible as well.
The decision to move versus copy data is important. For many organizations, data growth is reaching crisis proportions. Response times struggle to perform when datasets are too large. Batch processes may fail to complete in time, upending schedules. Downtime windows required for system upgrades may require extension. Storage costs are increased, and disaster recovery processes become even more challenging. A move process purges data at the source, relieving performance pressure on production systems, whereas a copy process increases infrastructure requirements by doubling the amount of data to process.
Conclusion
So, as data lakes roll out within your organization, remember that filling them may be the hardest part. An enterprise data lake with a federated big data governance model establishes a more reliable system of centralized compliance and enables decentralized data lakes to flourish.