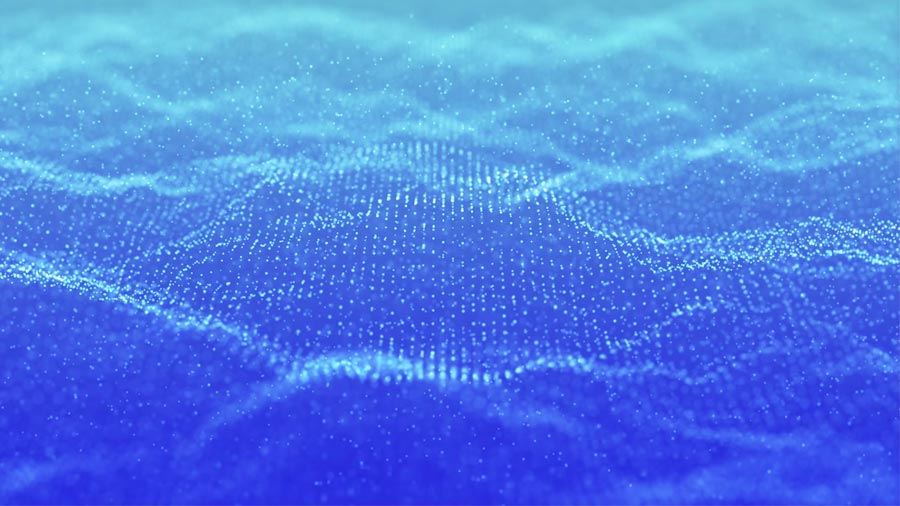
What is an Enterprise Data Lake?
The rise of multi-cloud, data-first architectures and the broad portfolio of advanced data-driven applications that have arrived as a result, rely on data lakes to store all the data. A data lake is an open source and industry standard repository for storing large amounts of data. Subsequently, an enterprise data lake not only stores data, they also provide enterprise grade services to collect, explore, manage, govern, prepare and build pipelines for enterprise data.
Enterprise data lakes either store data ‘as-is’ at the time of ingestion to avoid time-consuming and expensive ETL processes, or they provide data preparation services. These services profile, cleanse, enrich, transform, model and create data pipelines to meet specific application requirements. The goal is to enable real-time data-driven applications. Data preparation improves data quality and enables advanced analytics and business intelligence applications.
Data Pipelines for the Data-driven Enterprise
Data-driven applications leverage vast and complex networks of data and services. Enterprise data lakes deliver the connections necessary to move data from any source to any target location. Because they handle very large volumes of data and scale horizontally using commodity cloud infrastructure, enterprise data lakes are an ideal platform for cloud data migration, enterprise archiving, and Operational Data Store (ODS). Moreover, they have the capability to build pipelines between production systems and downstream analytics, SQL data warehouse, artificial intelligence (AI) and machine learning (ML) applications.
Data pipelines are a series of data flows. The output of one element is the input of the next one, and so on. Enterprise data lakes serve as the collection and access points in a data pipeline and are responsible for access control. As data pipelines emerge across the enterprise, enterprise data lakes become data distribution hubs with centralized controls to federate data across networks of data lakes. Data federation centralizes metadata management, data governance and compliance control while at the same time enabling decentralized data lake operations.
Of course managing data on such a large scale means data governance controls are essential. An enterprise data lake governs data with Information Lifecycle Management (ILM) policies. These establish a system of controls and business rules, including data retention policies and legal holds. Security and consumer data privacy controls like NIST 800-53, PCI, HIPAA, and GDPR are not only essential for legal compliance, proper implementation improves data quality as well.
Centralized Metadata Management
Enterprise data lakes need metadata management to view the entire data landscape (including structured, semi-structured, and unstructured data) and helps users understand their data better. Analysts classify, profile and establish consistent descriptions and business context for the data. Centralized metadata management enables users to explore their data landscape in three ways:
- Data lineage helps users understand the data lifecycle including a history of data movement and transformation. This simplifies root cause analysis by tracing data errors and improves confidence for processing by downstream systems.
- A data catalog is a portfolio view of data inventory and data assets. In other words, users browse the data that they need and are able to evaluate data for intended uses.
- Business Glossary is a list of business terms with their definitions. Data governance programs require that business concepts for an organization be defined and used consistently.
The centerpiece of cloud data management programs
Digital transformation requires interoperability with the cloud and its vast network of data and web services. Data lakes are an open source, industry standard approach to safely and securely collect and store large amounts of data. Additionally, an enterprise data lake provides enterprise grade services to explore, manage, govern, prepare and provide access control. Managers seeking these data-driven advantages therefore deploy enterprise data lakes to improve customer engagement, or provide improved analytics based on more complete, event-driven data.
In conclusion, data-first architectures require low-cost and efficient object storage, real-time access, data governance, metadata management, data preparation and connectivity to build end-to-end data pipelines. With an enterprise data lake, any organization is able to implement these critical capabilities very quickly, achieve digital transformation, and become a data-driven enterprise.